New Paper Published in Sensors Journal
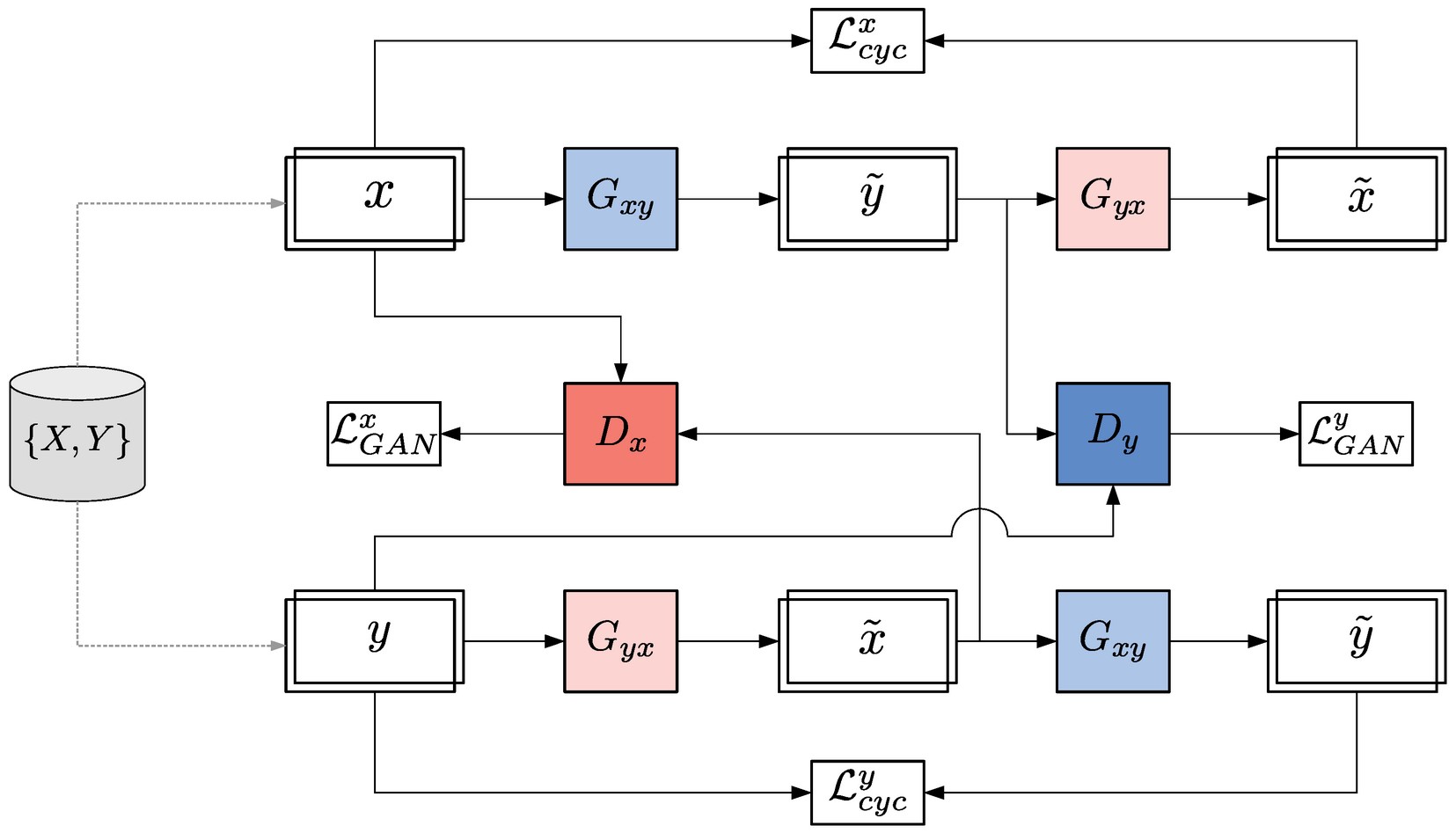
The previously mentioned paper was published in Sensors Journal on February 1st 2023, belonging to the Special Issue “Biosensors for Diagnostic Applications”. Nicolás is a PhD candidate in our group, who focuses in Deep Learning for Cardiovascular health estimation. We congratulate Nicolás on this amazing accomplishment.
The paper can be read here.
Central Arterial Dynamic Evaluation from Peripheral Blood Pressure Waveforms Using CycleGAN: An In Silico Approach
by Nicolás Aguirre, Leandro J. Cymberknop, Edith Grall-Maës, Eugenia Ipar and Ricardo L. Armentano
Arterial stiffness is a major condition related to many cardiovascular diseases. Traditional approaches in the assessment of arterial stiffness supported by machine learning techniques are limited to the pulse wave velocity (PWV) estimation based on pressure signals from the peripheral arteries. Nevertheless, arterial stiffness can be assessed based on the pressure–strain relationship by analyzing its hysteresis loop. In this work, the capacity of deep learning models based on generative adversarial networks (GANs) to transfer pressure signals from the peripheral arterial region to pressure and area signals located in the central arterial region is explored. The studied signals are from a public and validated virtual database. Compared to other works in which the assessment of arterial stiffness was performed via PWV, in the present work the pressure–strain hysteresis loop is reconstructed and evaluated in terms of classical machine learning metrics and clinical parameters. Least-square GAN (LSGAN) and Wasserstein GAN with gradient penalty (WGAN-GP) adversarial losses are compared, yielding better results with LSGAN. LSGAN mean ± standard deviation of error for pressure and area pulse waveforms are 0.8 ± 0.4 mmHg and 0.1 ± 0.1 cm2, respectively. Regarding the pressure–strain elastic modulus, it is achieved a mean absolute percentage error of 6.5 ± 5.1%. GAN-based deep learning models can recover the pressure–strain loop of central arteries while observing pressure signals from peripheral arteries.